新湖畔网 (随信APP) | 问答:如何缩小人工智能准备程度的差距
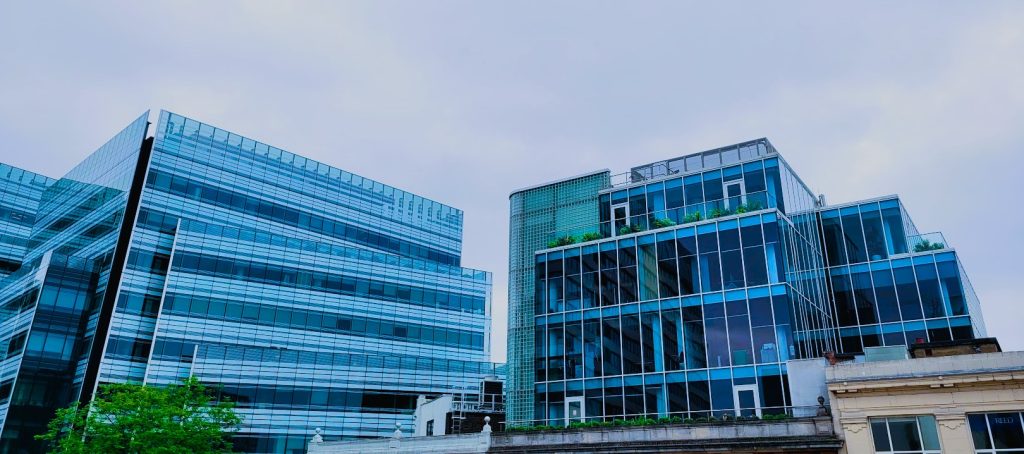
新湖畔网 (随信APP) | 问答:如何缩小人工智能准备程度的差距
【微信/公众号/视频号/抖音/小红书/快手/bilibili/微博/知乎/今日头条同步报道】
伦敦的商务单位。图像由Tim Sandle提供
组织必须跨越一座人工智能桥,才能完全采用生成式人工智能。为了了解这一点,Digital Journal采访了Sutherland Global的CIO兼首席数字官Doug Gilbert。
作为CIO和CDO,Gilbert定期监督产品和技术的发展和转型,具有通过部署技术增强客户体验和业务的良好记录。
Digital Journal: 从您的经验来看,一个健壮的数字基础设施和数据战略如何加快无缝整合人工智能?
Doug Gilbert:人工智能倡议经常无法扩展或交付有意义的结果,如果没有坚实的技术基础架构。强大的数字核心确保系统能够处理人工智能的高需求,从数据处理到与云环境的无缝集成,使组织能够有效扩展人工智能能力。
同样重要的是一个明确定义的数据战略。无论是利用混合、私有还是多云方法,安全和可访问的数据对于构建强大的人工智能解决方案至关重要。强大的数据基础不仅支持人工智能,还确保了全面合规和安全,在数据隐私至关重要的行业中尤为重要。
银行业是一个引人注目的例子,在这个领域,主要全球银行将人工智能整合到业务中以增强欺诈检测。通过确保他们的数字基础设施可以实时处理大量交易数据,并制定清晰的数据治理策略,银行大幅减少了欺诈检测所需的时间,同时保持监管合规性。这种转变之所以可能,是因为数字核心和数据架构对支持人工智能系统迅速准确地识别异常至关重要。
在这个方程中,人才的作用不容忽视。数据科学家和工程师是人工智能成功的关键,确保数据被收集、清洁和正确结构化以供人工智能模型使用。良好管理的数据使人工智能系统能够做出准确的预测,产生更有影响力的业务结果。
DJ: 您认为为什么一些高管在确定和衡量生成式人工智能采用的好处方面感到困难?
Gilbert:许多高管对生成式人工智能(Gen AI)在哪些地方可以提供最大价值感到不确定,特别是在一些行业中,使用案例仍在探索中。如果没有清晰理解它如何直接影响他们的具体业务挑战或流程,辨别好处就会困难。
此外,Gen AI的相对新颖性意味着许多组织缺乏内部专业知识来评估其全部潜力。高管可能不知道如何正确衡量成功,因为他们的团队仍在建立人工智能技能,或者严重依赖外部顾问。我认为高管应该首先确定受影响的领域,并持续测量它们。
例如,在零售业,公司利用Gen AI大规模创建个性化营销,通过生成个性化内容,如产品推荐和电子邮件,定制客户偏好。这导致参与率增加,在某些情况下,销售转化率提高了20%。通过不断跟踪参与率、购买转化率和客户终身价值等指标,高管可以清楚衡量Gen AI直接在推动收入增长和客户满意度方面的好处。
DJ: 基础设施和数据生态系统的差距对寻求人工智能准备性的企业造成了什么挑战?
Gilbert:人工智能准备度经常被忽视的一个方面是许多企业存在的重大基础设施和数据生态系统差距。为实现人工智能所需的基础设施需要大量资本和人才投资,往往达到数千万美元。没有这些投资,扩大人工智能工作变得困难。
人工智能对极大的计算能力和大量数据的需求通常会迫使组织朝着云迁移。然而,许多企业缺乏一致的云策略,这妨碍了他们处理人工智能工作负载所需的可扩展性和灵活性。强大的云基础设施确保数据能够在组织内流动,使人工智能模型能够实时处理、分析和提供见解。
此外,数据生态系统中的差距,如数据源集成不良或缺乏适当的数据治理,导致效率低下。人工智能模型依赖高质量、结构良好的数据进行训练和执行。没有坚实的数据基础,人工智能工作很可能会产生不可靠或不完整的结果,削弱了人工智能能为业务带来的潜在价值。
实质上,人工智能的成功不仅仅是关于技术本身,而是关于建立正确的基础设施和数据战略来支持它。
DJ: 您确定的关键技能短缺可能会使企业内的人工智能驱动倡议受阻?
Gilbert:一项2024年的调查表明,虽然81%的IT专业人员认为自己可以利用人工智能,但只有12%真正具备必要的技能。此外,70%的员工可能需要升级其人工智能技能,以在不断发展的领域保持竞争力。
以下是我观察到的可能会阻碍组织内人工智能倡议的几个技能差距:
● 数据科学和机器学习专业知识 - 公司经常很难找到具有深厚数据科学和机器学习专业知识的人才,因此,主动提升员工在这些领域的能力至关重要。没有这些技能,组织可能会难以开发和部署有效的人工智能技术,产生积极的业务结果。
● 数据工程和管理 - 拥有数据科学家很重要,也许更重要的是拥有能够有效和高效地管理公司在其人工智能技术中使用的数据的员工。没有有效的数据管理和工程,公司可能会面临人工智能项目和模型无效的风险。
● 伦理与人工智能治理 - 随着人工智能承担更多决策角色,缺乏专门团队监督道德人工智能使用的问题越来越成为一个问题。没有这些团队,公司可能会冒着将不道德或有偏见的人工智能模型部署到组织中的风险。麦肯锡指出,只有18%的组织建立了人工智能治理委员会,许多组织没有准备好应对与数据偏见、隐私或模型准确性相关的风险。
● 人工智能中的人类参与应用 - 虽然员工拥有技术人工智能技能是重要的,但同样重要的是培训员工将人类因素重新引入人工智能流程。确保人工智能程序与人类专业知识和决策协同工作,对于确保公司可以战略性地利用人工智能推动业务目标和胜利至关重要。
DJ: 公司如何主动确定和解决人工智能准备性差距,以防止项目价值流失并最大化回报?
Gilbert:要成功弥合人工智能准备性差距,公司需要一种有条理的方法。首先,系统评估技能水平是至关重要的。这可以通过客观的技能评估和员工的自我评估相结合来完成,两者进行比较以提供对现有能力和差距的准确理解。定期与行业标准进行对比可以进一步帮助确定工作人员滞后的领域。
除了技能评估,机构应该培养一个持续学习的文化,因为人工智能和相关技术发展迅速。提供持续的学习机会,如认证、实践工作坊和数字课程,将确保员工不断发展新技能。重要的是要认识到解决人工智能技能差距不是一个“一劳永逸”的过程;这需要不断承诺重新培训和提升技能。
另一个公司需要解决的重要方面是对变革的抵抗。这可以通过强调受益于提升技能努力的员工成功故事来实现。展示个人如何通过持续学习和适应人工智能增强了他们的职业生涯,可以激励其他人效仿。此外,将这些努力与有形的结果联系起来,如提高效率、创新或业务成功,强化随着时间的推移演进技能集的重要性。
此外,公司可以利用人工智能准备性框架,如麦肯锡或Gartner提供战略指导,确定基础设施、数据管理和人工智能治理方面的差距。通过对技能发展、数据基础设施和文化变革采取全面的方法,组织可以避免常见的陷阱,并成功地将人工智能整合到其运作中。
#桥接 #准备性 #差距
英文版:
Business units in London. Image by Tim Sandle
There is an AI bridge that organizations must cross to fully adopt generative AI across the enterprise. To learn about this, Digital Journal connected with Doug Gilbert, CIO and Chief Digital Officer at Sutherland Global.
As CIO and CDO, Gilbert regularly oversees product and technology development and transformation, with a proven track record of enhancing customer experiences and business through the deployment of technology.
Digital Journal: From your experience, how does a robust digital infrastructure and data strategy accelerate seamless AI integration?
Doug Gilbert: AI initiatives often struggle to scale or deliver meaningful results without a solid foundation of technology infrastructure. A strong digital core ensures that the systems can handle the high demands of AI, from data processing to seamless integration with cloud environments, allowing organizations to scale AI capabilities efficiently.
Equally important is a well-defined data strategy. Whether leveraging a hybrid, private, or multi-cloud approach, secure and accessible data is essential for building robust AI solutions. A strong data foundation not only supports AI but also ensures compliance and security across the board, which is crucial in industries where data privacy is paramount.
One compelling example is in the banking sector, where major global banks integrate AI into their operations to enhance fraud detection. By ensuring their digital infrastructure can manage vast volumes of transactional data in real time and developing a clear data governance strategy, the banks drastically reduce the time taken to fraud detection while maintaining regulatory compliance. This transformation is only possible because the digital core and data architecture are aligned to support AI systems in identifying anomalies swiftly and accurately.
The role of talent in this equation cannot be understated. Data scientists and engineers are pivotal to AI success, ensuring that data is collected, cleaned, and structured properly for AI models. Well-managed data allows AI systems to make accurate predictions, yielding more impactful business outcomes.
DJ: Why do you think some executives are struggling to identify and measure the benefits of generative AI adoption?
Gilbert: Many executives are uncertain about where Generative AI (Gen AI) can provide the most value, especially in industries where use cases are still being explored. Without a clear understanding of how it directly impacts their specific business challenges or processes, identifying benefits can be difficult.
Additionally, the relative newness of Gen AI means that many organizations lack in-house expertise to evaluate its full potential. Executives may not know how to properly measure success because their teams are still building AI skills or rely heavily on external consultants. Where I think an executive scan starts by identifying the areas of impact and continuously measuring them.
For instance, in retail, companies have used Gen AI to create personalized marketing at scale by generating individualized content, such as product recommendations and emails, tailored to customer preferences. This has resulted in increased engagement rates and, in some cases, a 20% rise in sales conversions. By continuously tracking metrics like engagement rates, purchase conversions, and customer lifetime value, executives can clearly measure the direct benefits of Gen AI in driving revenue growth and customer satisfaction.
DJ: What challenges do gaps in infrastructure and data ecosystem pose to enterprises aiming for AI readiness?
Gilbert: One often overlooked aspect of AI readiness is the significant infrastructure and data ecosystem gaps that exist in many enterprises. Building the necessary infrastructure for AI requires substantial capital and talent investment, often reaching tens of millions of dollars. Without these investments, scaling AI efforts becomes challenging.
AI's demand for immense computing power and vast amounts of data often forces organizations toward cloud migration. However, many enterprises lack a coherent cloud strategy, which hampers their ability to handle the scalability and flexibility AI workloads require. A robust cloud infrastructure ensures that data can flow seamlessly across the organization, enabling AI models to process, analyze, and deliver insights in real time.
Additionally, gaps in the data ecosystem, such as poorly integrated data sources or lack of proper data governance, lead to inefficiencies. AI models depend on high-quality, well-structured data for training and execution. Without a solid data foundation, AI efforts are likely to result in unreliable or incomplete outcomes, undermining the potential value AI can bring to the business.
In essence, AI success is not just about the technology itself but about having the right infrastructure and data strategies in place to support it.
DJ: What key skill shortages have you identified that could derail AI-driven initiatives within businesses?
Gilbert: A 2024 survey highlights that while 81% of IT professionals believe they can utilize AI, only 12% actually possess the necessary skills to do so. Furthermore, 70% of workers will likely need to upgrade their AI skills to stay competitive in the evolving landscape.
Here are several skills gaps that I've observed that could potentially hinder AI initiatives within organizations:
● Data Science and ML expertise – Companies often struggle to find talent that has deep data science and ML expertise, so proactively upskilling employees in these areas is of paramount importance. Without these skills, organizations may struggle to develop and deploy effective AI technologies that generate positive business results.
● Data Engineering and Management – While having data scientists is important, perhaps more important is having employees who can effectively and efficiently manage the data companies use in their AI technologies. Without effective data management and engineering, companies can run the risk of having ineffective AI programs and models.
● Ethics + AI Governance—As AI takes on more decision-making roles, the absence of dedicated teams to oversee ethical AI usage is increasingly becoming a concern. Without them, companies could risk deploying unethical and/or biased AI models into an organization. McKinsey notes that only 18% of organizations have established AI governance boards, and many organizations are unprepared to tackle risks related to data bias, privacy, or model accuracy.
● Human in the Loop Applications of AI—While possessing technical AI skills is important for employees, it's also essential that companies train employees to introduce the human element back into AI processes. Ensuring that AI programs work symbiotically with human expertise and decision-making is critical to ensuring companies can strategically use AI to drive forward business goals and wins.
DJ: How can companies proactively pinpoint and address AI readiness gaps to prevent project values and maximize returns?
Gilbert: To successfully bridge gaps in AI readiness, companies need a structured approach. First, systematically assessing skill levels is crucial. This can be done through a combination of objective skills assessments and self-evaluations by employees, with the two being compared to provide an accurate understanding of existing competencies and gaps. Regular benchmarking against industry standards can further help identify areas where the workforce is lagging.
Beyond skill assessments, organizations should foster a culture of continuous learning as AI and related technologies evolve rapidly. Providing ongoing learning opportunities—such as certifications, hands-on workshops, and digital courses—will ensure employees are consistently developing new skills. It's important to recognize that addressing the AI skills gap is not a "one-and-done" process; it requires an ongoing commitment to reskilling and upskilling.
Another important aspect that companies need to address is resistance to change. This can be done by highlighting success stories of employees who have benefited from upskilling efforts. Showcasing how individuals have enhanced their careers through continuous learning and adapting to AI can inspire others to follow suit. Additionally, linking these efforts to tangible outcomes—like improved efficiency, innovation, or business success—reinforces the importance of evolving skill sets over time.
Moreover, companies can leverage AI readiness frameworks, such as those from McKinsey or Gartner, which provide strategic guidance for identifying gaps in infrastructure, data management, and AI governance. Organizations can avoid common pitfalls and successfully integrate AI into their operations by taking a comprehensive approach to skills development, data infrastructure, and cultural change.
Q&A: Bridging the AI readiness gap
#Bridging #readiness #gap
关注流程:打开随信App→搜索新湖畔网随信号:973641 →订阅即可!
公众号:新湖畔网 抖音:新湖畔网
视频号:新湖畔网 快手:新湖畔网
小红书:新湖畔网 随信:新湖畔网
百家号:新湖畔网 B站:新湖畔网
知乎:新湖畔网 微博:新湖畔网
UC头条:新湖畔网 搜狐号:新湖畔网
趣头条:新湖畔网 虎嗅:新湖畔网
腾讯新闻:新湖畔网 网易号:新湖畔网
36氪:新湖畔网 钛媒体:新湖畔网
今日头条:新湖畔网 西瓜视频:新湖畔网