新湖畔网 (随信APP) | 别人刚学习如何使用"端到端"技术,但理想智驾却已经更新换代了。
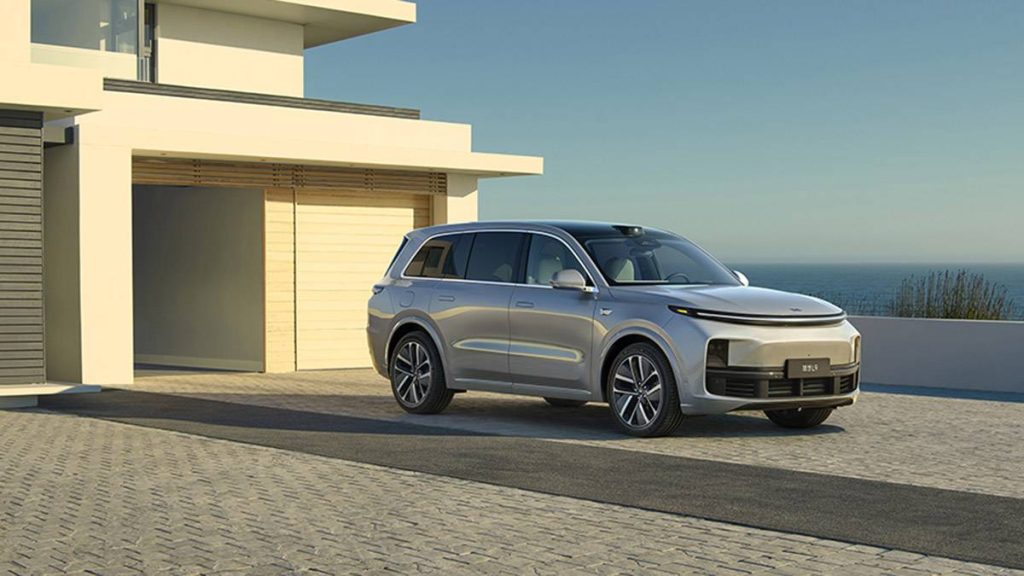
新湖畔网 (随信APP) | 别人刚学习如何使用"端到端"技术,但理想智驾却已经更新换代了。
【微信/公众号/视频号/抖音/小红书/快手/bilibili/微博/知乎/今日头条同步报道】
自动驾驶技术发展了这么多年,最大的变化是什么?
在写下这篇文章的前一天,两位汽车行业朋友来到爱范儿,和我们坐下聊了聊。聊到的内容有很多,从产品推广到行业趣闻,而自动驾驶作为行业热议的一个分支,自然也成为了我们讨论的焦点之一。
回顾自动驾驶这些年来的发展,变化其实有不少,包括传感器的迭代、车端算力的提升、从高精地图过渡到占用网络等。但在这些变化中,最引人注目的突破当属大模型的加入。
大模型,让自动驾驶技术的应用,变得触手可及。
10 月 23 日,理想汽车全新一代双系统智能驾驶解决方案「端到端+VLM」正式开始全量推送,理想汽车的智能驾驶,从此步入了 AI 大模型的时代。
像人一样思考,像人一样驾驶,如今的理想汽车,正在实现这一愿景。
好不容易搞懂了端到端,VLM 又是什么?
关于端到端到底是什么?是从哪个「端」到哪个「端」?别说普通消费者了,就连不少媒体从业者都没有搞清楚。
不少厂商都曾对此做出过解释,其中解释得最通俗易懂的,还是理想汽车:
一端,是传感器:摄像头、激光雷达等传感器,它们就像是人的眼睛,负责输入环境信息。此外还有特别设计的输入信息,如车辆的位置、位姿和导航等信息。
另一端,是行驶轨迹:接收了来自传感器的信息后,系统会输出「动态障碍物」、「道路结构」、「占用网络 Occ」和「规划轨迹」。前三个感知任务主要通过屏幕呈现给用户,第四个「行驶轨迹」,就是我们最终需要从传感器映射出来的东西。
不难发现,从传感器接收信息,到系统输出行驶轨迹这个过程,和我们自己开车非常类似——我们的眼睛负责接收信息,双手会自然而然地带动方向盘,把车辆带到正确的轨迹上。
是的,依靠端到端模型,理想新一代智驾系统做到了像人一样驾驶。
一直以来,无论是主机厂还是自动驾驶企业,都在不断宣传自家的智驾系统有多么类人,多么像「老司机」。然而,一些「老司机」们习以为常的场景,在很长一段时间里,都是难以解决的行业难题。
最典型的就是环岛这一场景,因为场景复杂、感知受限,因此在今年 7 月之前,还没有几家车企能够实现「老司机」般的进出环岛。
理想智驾技术研发负责人贾鹏曾对爱范儿和董车会表示,对于感知和规控分离的分段式智驾方案来说,在环岛场景里,感知模型需要为规控模型做「各种各样的假设。」
做个掉头,还得把掉头线拟合出来,不同的路口的掉头还不太一样,曲率都不太一样,所以你很难做到一套代码就可以把所有环岛掉头搞定,种类太多了。
一体式的端到端方案则不同,其具备更强的复杂道路结构的理解能力,可以运用人类驾驶员数据训练出不同的环岛类型、不同出入口的进出轨迹,自主选择合适的行进路线。
如此一来,原有的道路拓扑和人工定义的规则,就再是必须的了。
关于环岛这件事,贾鹏还分享过一个「好玩的故事」。
在我们(的模型数据包含)大概 80 万 clips(视频片段)的时候,还过不了环岛,后来突然有一天发现我们(喂了)100 万 Clips(之后)它自己能过环岛,我觉得是 100 万(视频片段)里头刚好有一些环岛数据放在里面了。
「模型确实很厉害,」贾鹏补充道,「你喂了什么数据他就能学会,这是模型的魅力所在。」
理想如今推出的全量版本基于 V4.8.6 模型,后者是在 400 万 clips 的基础上迭代的第 16 个版本。和以往相比,新模型对于超车场景和导航信息的理解能力得到提升,同时,障碍物的检测更加精准,绕行的幅度也更为合理。
因此不仅是环岛,像 U 型掉头、拥堵时的蠕行和博弈、十字路口等传统复杂场景,如今的「端到端+VLM」智驾系统,都能够很好地自主处理,甚至还支持 P 档激活——
在路边停车时,用户原地双击拨杆来激活智驾系统,不必再像以前一样,必须在车道内才能激活。
介绍完端到端模型的能力,接下来就是 VLM 模型。
VLM 模型是一种视觉语言模型,理想是第一个将视觉语言模型成功部署在车端芯片的厂商,使自动驾驶具备了未知场景的逻辑思考能力。
也就是说,它能够像人一样思考。
举个例子,能够生成行驶轨迹的端到端模型,完全具备通过收费站的能力,但它在面对收费站时,并不是很清楚自己应该走哪条道,最后只能随便挑一条来走。
而 VLM 模型,则能够像人类一样理解物理世界的复杂交通环境和中文语义,可以清楚地分辨 ETC 车道和人工车道,并辅助端到端模型做出正确的决策。
类似的场景其实还有很多,如公交车道和潮汐车道的识别、学校路段等路牌的识别、主辅路的进出等。不仅如此,在遇到施工场景、坑洼路面甚至是减速带时,VLM 模型也能很好地理解,进行提醒和降速。
截至目前,理想汽车的 VLM 视觉语言模型已经拥有了 22 亿的参数量,对物理世界的复杂交通环境具有更拟人的理解能力。
此外,在 OTA 6.4 版本中,高速 NOA 功能也得到了优化,在高速 &城市快速路场景中,系统可以更早地识别前方慢车,超车动作更加高效安全。
总而言之,在端到端+VLM 双系统的帮助下,如今面向用户的 OTA 6.4,其拟人化程度上到了一个新的台阶。
理想的「快」与「慢」
从技术架构来看,理想汽车这两年经历了三次比较大的调整。
从需要先验信息的 NPN 网络,再到基于 BEV 和占用网络的无图 NOA,再到如今的一体化端到端技术路线。
第一代 NPN 架构比较复杂,包含了感知、定位、规划、导航、NPN 等模块,它们共同支撑起了理想汽车当时 100 城的城市 NOA 推送。
第二代无图 NOA,理想汽车引入了端到端大模型,模块数量大幅缩减,只剩下了感知和规划,不再需要等待先验信息的更新。
理想的这一步,让车企的「卷」,不再局限于无聊的开城数量,真正实现了有导航就能开。
今年 5 月,理想汽车招募了 1000 位用户,正式开启了无图 NOA,也就是 AD Max 3.0 的公测。当时的用户反馈,远远超出了理想汽车的预期,短短两个月后,理想汽车就为 24 万多位理想 AD Max 用户推送了这次升级。
只不过,这个时候的端到端,还是一个分段式的端到端,第三代智驾方案,才是真正意义上的一体式端到端——从输入到输出,全部由一个模型实现,中间没有任何规则的参与。
在以往,无论是有图方案还是无图方案,都依赖工程师根据各种各样的道路场景去编写规则,力图穷举所有道路状况和与之对应的方案,让智驾的范围尽可能地广。
通常来说,厂商会把场景大致分为三种:高速场景、城区场景和泊车场景。这几大场景又可以继续细分,规控工程师们则需要针对这些场景来编写代码。
但面对错综复杂的现实世界,这样的做法显然不够现实。而一体式端到端,则可以学习人类开车的过程,接收传感器信息后,直接输出行驶轨迹。
有没有发现,这个时候,提升智驾能力最重要的因素,从工程师变成了数据。而理想,最不缺的就是数据。
10 月 14 日,理想汽车迎来了第 100 万辆整车在江苏省常州基地下线,中国首个百万辆新势力车企就此诞生。根据理想汽车公布的数据,在 30 万元以上的理想车型中,AD Max 用户的比例,高达 70%——
每过一个月,这些车都能给理想提供十几亿公里的训练数据。
另外,理想很早就意识到数据的重要意义,打造了关于数据的工具链等基础能力,比如理想的后台数据库实现了一段话查找当时,写一句「雨天红灯停止线附近打伞路过的行人」,就能找到相应的数据。
正是凭借庞大的训练数据和完善的控制链,理想智驾实现了在行业中的「后来居上」,用端到端和 VLM 组成了自己的「快」与「慢」。
在理想看来,这套双系统智驾方案,类似于诺贝尔奖获得者丹尼尔·卡尼曼在《思考,快与慢》中的快慢系统理论:
人的快系统依靠直觉和本能,在 95% 的场景下保持高效率;人的慢系统依靠有意识的分析和思考,介绍 5% 场景的高上限。
其中,端到端是那个「快系统」,而 VLM 自然就是「慢系统」了。
郎咸朋认为,一个自动驾驶系统到底是 L3 级别还是 L4 级别,并不取决于端到端,VLM 模型才是真正能去应对未知场景,拔高能力上限的关键所在。
理想的「快」与「慢」
从技术架构来看,理想汽车这两年经历了三次比较大的调整。
从需要先验信息的 NPN 网络,再到基于 BEV 和占用网络的无图 NOA,再到如今的一体化端到端技术路线。
第一代 NPN 架构比较复杂,包含了感知、定位、规划、导航、NPN 等模块,它们共同支撑起了理想汽车当时 100 城的城市 NOA 推送。
第二代无图 NOA,理想汽车引入了端到端大模型,模块数量大幅缩减,只剩下了感知和规划,不再需要等待先验信息的更新。
理想的这一步,让车企的「卷」,不再局限于无聊的开城数量,真正实现了有导航就能开。
今年 5 月,理想汽车招募了 1000 位用户,正式开启了无图 NOA,也就是 AD Max 3.0 的公测。当时的用户反馈,远远超出了理想汽车的预期,短短两个月后,理想汽车就为 24 万多位理想 AD Max 用户推送了这次升级。
只不过,这个时候的端到端,还是一个分段式的端到端,第三代智驾方案,才是真正意义上的一体式端到端——从输入到输出,全部由一个模型实现,中间没有任何规则的参与。
在以往,无论是有图方案还是无图方...
「理想同学,我要去这里」
除了智能驾驶方面的升级,OTA 6.4 在用户交互方面也引来了革新。
这里同样分为「快」和「慢」两个部分。
作为「快系统」的端到端模型所对应的通常为文字弹窗,为驾驶员实时提供导航
英文版:
What has been the biggest change in the development of autonomous driving technology over the years?
Two friends from the automotive industry came to iFanr the day before this article was written and sat down to chat with us. We discussed many topics, from product promotion to industry anecdotes, and autonomous driving, as a hot topic in the industry, naturally became one of our focal points.
Looking back on the development of autonomous driving over the years, there have been many changes, including iterations of sensors, improvement of on-board computing power, transitioning from high-precision maps to network occupancy, and more. But among these changes, the most notable breakthrough is undoubtedly the introduction of large models.
Large models have made the application of autonomous driving technology more accessible.
On October 23rd, Ideal Auto's new generation dual-system intelligent driving solution "End-to-End+VLM" officially began full-scale deployment, marking the entry of Ideal Auto's intelligent driving into the era of AI large models.
Thinking like a human, driving like a human, today's Ideal Auto is realizing this vision.
Just when we understood end-to-end, what is VLM?
What exactly is end-to-end? From which "end" to which "end"? Even many media professionals have not fully understood it.
Many companies have tried to explain this, with Ideal Auto offering the most accessible explanation:
One end is the sensors: cameras, LiDAR, and other sensors, which are like the eyes of a person, responsible for inputting environmental information. Additionally, there are specifically designed input information, such as the vehicle's position, posture, navigation, and other information.
The other end is the driving trajectory: after receiving information from the sensors, the system outputs "dynamic obstacles," "road structure," "network occupancy Occ," and "planned trajectory." The first three perception tasks are mainly presented on the screen to the user, and the fourth "driving trajectory" is what we ultimately need to map out from the sensors.
It is easy to see that the process from the sensors receiving information to the system outputting the driving trajectory is very similar to our own driving process - our eyes are responsible for receiving information, and our hands naturally guide the steering wheel to put the vehicle on the correct trajectory.
Yes, relying on the end-to-end model, Ideal's new generation intelligent driving system has achieved driving like a human.
Both the main car companies and autonomous driving enterprises have been constantly promoting their intelligent driving systems as being very human-like, similar to the "old drivers." However, some of the scenarios that these "old drivers" are accustomed to have been hard-to-solve industry problems for a long time.
The most typical example is the roundabout scenario, which, due to its complexity and limited perception, few vehicle companies were able to achieve "old driver" level entry and exit from roundabouts before July of this year.
Jia Peng, head of Ideal's smart driving technology research and development, once told iFanr and TMM about the segmented smart driving solution with separated perception and rule control that in the roundabout scenario, the perception model needs to make "various assumptions" for the rule control model.
For example, making a U-turn, you also have to fit the U-turn line, different intersections have different U-turns, different curvatures, so it's hard for you to come up with a set of codes that can handle all roundabout U-turns, there are too many variations.
In contrast, the integrated end-to-end solution has a stronger understanding of complex road structures, can train different roundabout types and entrance and exit trajectories using human driver data, and autonomously select suitable travel routes.
As a result, the original road topology and manually defined rules are no longer necessary.
About the roundabout situation, Jia Peng once shared a "fun story."
When our model data reached about 800,000 clips, it still couldn't pass through the roundabout. Later, one day we suddenly found that when we fed it 1 million clips, it could go through the roundabout on its own. I think that within the 1 million (video clips), there happened to be some roundabout data included.
"
The model is indeed very powerful," Jia Peng added, "It can learn whatever data you feed it, and that's the charm of the model."
The full version introduced by Ideal today is based on the V4.8.6 model, which is the 16th iteration based on 4 million clips. Compared to before, the new model has improved its understanding capability for overtaking scenarios and navigation information. Furthermore, obstacle detection is more accurate, and the detour is more reasonable.
As a result, not only roundabouts, but also traditional complex scenarios such as U-turns, creeping and bargaining in congestion, and intersections can now be handled autonomously by the "End-to-End+VLM" intelligent driving system, even supporting P-gear activation -
When parking on the roadside, users can activate the smart driving system by double-clicking the lever in place, no longer requiring activation only within the lane as before.
After introducing the capabilities of the end-to-end model, the next step is the VLM model.
The VLM model is a visual language model. Ideal is the first company to successfully deploy a visual language model on the vehicle chip, giving autonomous driving the ability to logicall...
If you want to read the full text, please visit: [原文链接](https://www.ifanr.com/1603707)
别人刚上「端到端」,理想智驾却又迭代了
#别人刚上端到端理想智驾却又迭代了
关注流程:打开随信App→搜索新湖畔网随信号:973641 →订阅即可!
公众号:新湖畔网 抖音:新湖畔网
视频号:新湖畔网 快手:新湖畔网
小红书:新湖畔网 随信:新湖畔网
百家号:新湖畔网 B站:新湖畔网
知乎:新湖畔网 微博:新湖畔网
UC头条:新湖畔网 搜狐号:新湖畔网
趣头条:新湖畔网 虎嗅:新湖畔网
腾讯新闻:新湖畔网 网易号:新湖畔网
36氪:新湖畔网 钛媒体:新湖畔网
今日头条:新湖畔网 西瓜视频:新湖畔网